Discover how the field of radiology is undergoing a transformative radiology revolution through the integration of AI technology. Explore how artificial intelligence is empowering radiologists to enhance their diagnostic abilities, improve efficiency, and detect medical conditions at an early stage. Learn about the benefits, challenges, and potential impact of this innovative collaboration between human expertise and advanced AI algorithms in the realm of medical imaging and diagnosis.
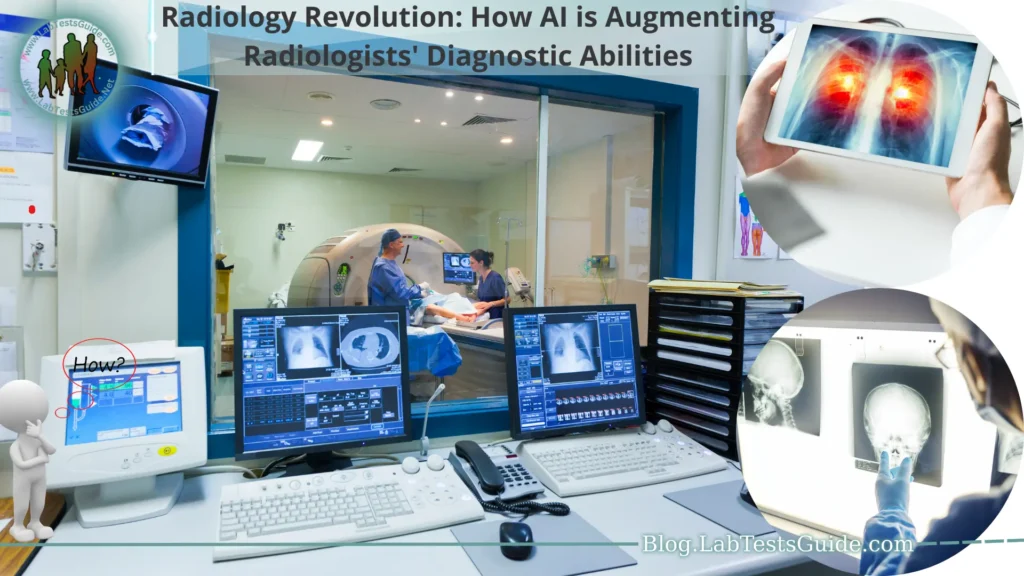
What is Radiology’s AI Revolution?
- Introduction to AI Revolution: Understand the paradigm shift brought by the integration of artificial intelligence (AI) into the field of radiology.
- AI in Medical Imaging: Explore how AI is transforming the interpretation of medical images, aiding radiologists in diagnosis.
- Enhanced Accuracy and Efficiency: Learn how AI algorithms can analyze vast amounts of data quickly and accurately, potentially reducing human error and enhancing efficiency.
- Anomaly Detection and Early Diagnosis: Discover how AI is being used to detect subtle anomalies and abnormalities that might be missed by human interpretation alone.
- Personalized Patient Care: Explore the potential for AI to enable personalized treatment plans by analyzing individual patient data and medical history.
- Improved Workflow: Understand how AI integration can streamline the radiologist’s workflow, allowing them to focus more on complex cases.
- Challenges and Considerations: Delve into the ethical, legal, and collaboration-related challenges posed by AI adoption in radiology.
- Human-AI Synergy: Recognize the importance of a collaborative approach, where radiologists work alongside AI to achieve the best outcomes.
- Future Prospects: Look ahead to the evolving role of AI in radiology and the possibilities for further advancements in diagnostic capabilities.
- Conclusion: Sum up the AI revolution’s impact on radiology and set the tone for the subsequent sections.
How to Embrace AI for Radiologists’ Enhanced Diagnostics:
- Understanding AI Basics: Begin by gaining a foundational understanding of what artificial intelligence (AI) is and how it can be applied to radiology.
- Educational Resources: Seek out specialized courses, workshops, and online resources that offer training in AI concepts and applications relevant to radiology.
- Collaboration with Data Scientists: Foster collaboration between radiologists and data scientists to develop and fine-tune AI algorithms tailored to medical image analysis.
- Accessing Quality Datasets: Ensure access to diverse and high-quality medical image datasets that are essential for training AI models effectively.
- Exploring AI Tools: Research and evaluate AI-powered radiology tools and software available in the market, considering factors like accuracy, user interface, and integration capabilities.
- Pilot Projects: Initiate small-scale pilot projects within your radiology department to test and validate the effectiveness of AI algorithms in real-world scenarios.
- Continuous Learning: Stay updated with the latest developments in AI and radiology by participating in conferences, webinars, and forums dedicated to medical AI applications.
- Ethical Considerations: Familiarize yourself with the ethical implications of AI in radiology, such as patient privacy, consent, and transparency in decision-making.
- Data Security and Compliance: Implement robust data security measures to protect patient information and ensure compliance with relevant regulations like HIPAA.
- Interdisciplinary Collaboration: Collaborate with clinicians, technologists, and other healthcare professionals to ensure a holistic approach to integrating AI into diagnostics.
- User Feedback and Adaptation: Solicit feedback from radiologists and healthcare staff using AI tools to continuously improve their functionality and address any concerns.
- Monitoring and Validation: Regularly monitor the performance of AI algorithms and validate their accuracy to maintain the quality of diagnostic outcomes.
- Cultural Shift and Training: Facilitate a cultural shift within the radiology department by providing training and support to radiologists adapting to AI-augmented diagnostics.
- Long-Term Strategy: Develop a long-term strategy for AI integration, considering factors like scalability, resource allocation, and the evolving landscape of medical AI.
- Embracing Change: Embrace AI as a complementary tool that can enhance diagnostic accuracy and efficiency, rather than a replacement for radiologists.
- Measuring Impact: Establish key performance indicators (KPIs) to measure the impact of AI on diagnostic accuracy, patient outcomes, and overall radiology workflow.
- Adapting to Advances: Stay open to advancements in AI technology and be prepared to adapt your strategies as the field evolves.
- Conclusion: Summarize the essential steps to effectively embrace AI for enhanced diagnostic capabilities, emphasizing the importance of continuous learning and collaboration.
The Emergence of AI in Radiology:
- Introduction to AI in Radiology: Introduce the concept of artificial intelligence (AI) and its increasing role in revolutionizing the field of radiology.
- Historical Context: Provide a brief overview of the historical development of AI technologies and their applications in medical imaging.
- Early Applications: Highlight some of the early use cases of AI in radiology, such as computer-aided detection (CAD) systems and automated image analysis.
- Advantages of AI: Discuss the advantages that AI brings to radiology, including its ability to analyze large datasets, detect subtle abnormalities, and assist radiologists in making accurate diagnoses.
- Machine Learning and Deep Learning: Explain the fundamental concepts of machine learning and deep learning, and how these techniques are driving the advancements in AI applications for radiology.
- Data-Driven Insights: Describe how AI algorithms learn from massive amounts of medical imaging data, enabling them to recognize patterns and anomalies that might elude human perception.
- Improved Efficiency: Discuss how AI can streamline radiologists’ workflow by automating repetitive tasks, allowing them to focus on more complex and critical cases.
- Challenges and Limitations: Address the challenges associated with AI adoption in radiology, including the need for high-quality labeled datasets, potential biases, and the importance of maintaining human oversight.
- Integration with Clinical Workflow: Explain how AI technologies are integrated into existing clinical workflows, including picture archiving and communication systems (PACS) and radiology information systems (RIS).
- Regulatory and Ethical Considerations: Touch upon the regulatory frameworks and ethical considerations that surround the use of AI in medical diagnosis, such as FDA approvals and patient privacy.
- Collaboration between Radiologists and AI: Emphasize the importance of collaboration between radiologists and AI systems, highlighting the symbiotic relationship between human expertise and machine capabilities.
- Impact on Radiology Education: Discuss how the emergence of AI is influencing the training and education of future radiologists, with a focus on understanding AI technologies.
- The Path Forward: Conclude the section by highlighting the potential for AI to continue evolving and shaping the landscape of radiology, with implications for patient care and research.
AI Applications in Radiology:
- Introduction to AI Applications: Introduce the diverse range of applications where artificial intelligence (AI) is making a significant impact in the field of radiology.
Image Recognition and Interpretation:
- Explain how AI algorithms can analyze medical images, including X-rays, CT scans, and MRIs, to assist radiologists in identifying anatomical structures and abnormalities.
- Highlight the use of convolutional neural networks (CNNs) and other deep learning techniques for image recognition.
Detection of Anomalies and Abnormalities:
- Discuss how AI is being used to detect subtle anomalies and early signs of diseases that might be challenging for human radiologists to identify.
- Provide examples of AI applications in detecting conditions like lung cancer, brain tumors, and cardiovascular issues.
Quantitative Analysis and Measurements:
- Explain how AI algorithms can provide precise quantitative measurements from medical images, such as tumor size, volume, and growth rates.
- Showcase the benefits of AI-enhanced measurements in tracking disease progression and treatment effectiveness.
Automated Report Generation:
- Describe how AI can assist in generating preliminary radiology reports by extracting relevant information from medical images and patient data.
- Highlight the potential for reducing radiologists’ reporting workload and improving report consistency.
Risk Stratification and Treatment Planning:
- Discuss how AI-powered predictive models analyze patient data to assess risks, predict outcomes, and aid in personalized treatment planning.
- Mention the use of AI in identifying patients who might benefit from specific interventions.
- Natural Language Processing (NLP) in Radiology: Explore how NLP techniques enable AI systems to analyze and understand written or spoken radiology reports, facilitating data extraction and insights.
Integrating AI with PACS and RIS Systems:
- Explain the integration of AI tools with picture archiving and communication systems (PACS) and radiology information systems (RIS) for seamless workflow enhancement.
- Real-time Decision Support: Discuss the potential for AI to provide real-time decision support to radiologists during image interpretation, suggesting potential diagnoses and treatment options.
Diagnostic Assistance and Second Opinions:
- Describe how AI can serve as a valuable tool for radiologists, offering diagnostic suggestions and acting as a “second opinion” system.
- Beyond Diagnostics: AI in Research: Highlight the use of AI in medical research, including drug discovery, disease modeling, and population health analysis using imaging data.
Patient-Focused Applications:
- Discuss AI applications that empower patients, such as AI-driven patient education tools and apps that help patients understand their medical images and reports.
- Future Possibilities: Conclude the section by touching on the limitless potential of AI applications in radiology, with advancements in AI technology opening doors to new and unforeseen possibilities.
Benefits and Opportunities of AI-Augmented Radiology:
- Introduction to AI-Augmented Radiology Benefits: Set the stage by introducing the myriad advantages and opportunities that arise from the integration of artificial intelligence (AI) into radiology practices.
Enhanced Accuracy and Precision:
- Explain how AI algorithms can analyze vast amounts of medical imaging data quickly and accurately, reducing the potential for human errors and oversights.
- Provide examples of AI’s ability to detect subtle abnormalities that might go unnoticed by human radiologists.
Efficiency and Workflow Improvement:
- Discuss how AI-powered automation can streamline routine tasks, such as image preprocessing and report generation, allowing radiologists to focus more on complex cases.
- Highlight the potential for faster turnaround times in reporting, leading to quicker patient care decisions.
- Improved Detection Rates: Describe how AI’s high sensitivity to patterns and anomalies can lead to improved detection rates for conditions like cancer, enabling earlier interventions and potentially better outcomes.
Personalized Treatment Plans:
- Explain how AI can analyze patient data to provide insights into personalized treatment plans based on individual medical histories and characteristics.
- Discuss the potential for tailoring interventions to optimize patient outcomes.
- Handling Data Overload: Address the challenge of managing the ever-increasing volume of medical imaging data and how AI can assist in processing and prioritizing this data.
- Reduced Radiologist Fatigue: Discuss how AI can alleviate radiologist fatigue by taking on repetitive tasks, allowing them to focus on critical thinking and complex analysis.
- Education and Skill Enhancement: Highlight the opportunities for radiologists to enhance their skills by learning to collaborate effectively with AI tools, resulting in a broader skill set.
- Remote and Telemedicine Applications: Explore how AI-augmented radiology can facilitate remote consultations and telemedicine, enabling expert opinions in areas with limited access to specialists.
- Advancing Research and Clinical Trials: Explain how AI can accelerate medical research by efficiently analyzing large datasets, contributing to advancements in understanding diseases and developing new treatments.
- Unlocking Imaging Insights: Discuss how AI can uncover insights from medical images that might not be immediately apparent to the human eye, leading to new discoveries and insights.
- Enhanced Patient Engagement: Describe how AI-powered visualizations and explanations can help patients understand their diagnoses and treatment plans, fostering better patient engagement.
- Economic Impact and Cost Savings: Address the potential economic benefits of AI-augmented radiology, such as reduced healthcare costs due to early detection and optimized treatment pathways.
Notable AI-Powered Radiology Tools:
- Introduction to AI-Powered Radiology Tools: Introduce the significance of AI-powered tools in radiology and their potential to revolutionize diagnostic practices.
Case Studies of Successful Implementations:
- Provide real-world examples of AI-powered radiology tools that have been successfully integrated into healthcare institutions.
- Highlight specific use cases, such as early cancer detection, fracture identification, or brain anomaly detection.
Overview of Leading AI Companies in Radiology:
- Briefly introduce prominent companies and startups that are developing cutting-edge AI solutions for radiology.
- Mention their key products, technologies, and notable partnerships.
User Experience and Radiologist Feedback:
- Discuss the experiences of radiologists and healthcare professionals who have used AI-powered tools in their daily practices.
- Include feedback on how these tools have impacted efficiency, accuracy, and patient care.
- Diagnostic Accuracy and Clinical Validations: Highlight instances where AI tools have demonstrated high diagnostic accuracy through rigorous clinical validations and comparisons with human experts.
- Workflow Integration and User-Friendly Interfaces: Describe how AI tools are designed to seamlessly integrate into existing radiology workflows, providing user-friendly interfaces that enhance radiologists’ experiences.
- Automated Anomaly Detection and Reporting: Explain how certain AI tools can automatically flag anomalies and generate preliminary reports, aiding radiologists in their decision-making process.
- AI-Assisted Quantitative Analysis: Discuss tools that enable AI-assisted measurements and quantitative analysis, providing consistent and precise measurements in various medical imaging modalities.
- Radiologist and AI Collaboration Models: Explore the different models of collaboration between radiologists and AI tools, emphasizing the importance of radiologists’ expertise in validating and interpreting results.
- Continual Learning and Model Updates: Address how AI tools evolve through continuous learning and updates based on new data and insights, ensuring their relevance and accuracy over time.
- Ethical Considerations and Patient Privacy: Discuss the ethical considerations associated with AI-powered radiology tools, including patient privacy, data security, and the responsible use of technology.
- Regulatory Approvals and Standards: Mention any regulatory approvals or standards that AI tools need to adhere to in order to ensure their safe and effective use in clinical settings.
- Future Directions and Evolving Capabilities: Conclude the section by discussing the potential future directions of AI-powered radiology tools, including advancements in AI algorithms, integration with other technologies, and expanding applications.
Ensuring a Smooth Transition:
- Introduction to Transitioning to AI: Introduce the concept of transitioning to AI-augmented radiology and the importance of a seamless integration process.
Training Radiologists in AI Integration:
- Discuss the need for comprehensive training programs that equip radiologists with the skills to effectively collaborate with AI tools.
- Highlight the importance of understanding AI concepts, interpreting AI-generated insights, and validating results.
Addressing Resistance to Technological Change:
- Explore common concerns and resistance that radiologists may have towards AI adoption.
- Provide strategies to address resistance through education, open communication, and emphasizing AI as a tool to enhance expertise.
Establishing Guidelines and Best Practices:
- Discuss the importance of establishing guidelines for the responsible and ethical use of AI in radiology.
- Highlight the need for standardized protocols, data quality assessments, and transparency in decision-making.
Human-AI Collaboration and Trust Building:
- Explain the dynamics of human-AI collaboration and how to build trust between radiologists and AI systems.
- Discuss the role of transparency, explainability, and providing insights into how AI arrives at its conclusions.
- Data Quality and Preprocessing: Address the significance of high-quality data for training AI algorithms and the importance of proper data preprocessing to ensure accurate results.
Interpreting AI Outputs and Validating Results:
- Provide guidance on how radiologists can effectively interpret AI-generated outputs, critically evaluate results, and validate findings against clinical context.
- Clinical Oversight and Decision-Making: Discuss the role of radiologists in maintaining clinical oversight, making final diagnostic decisions, and using AI as a complementary tool.
Risk Management and Error Mitigation:
- Explore strategies for managing risks associated with AI errors, including having contingency plans and mechanisms for identifying and rectifying mistakes.
- Continuous Learning and Adaptation: Emphasize the need for radiologists to engage in continuous learning as AI technologies evolve, staying updated on new features and improvements.
Collaboration with IT and Technical Teams:
- Discuss the importance of collaboration between radiology departments and IT/technical teams for successful AI integration and system maintenance.
- Feedback Mechanisms and Improvements: Highlight the value of radiologists’ feedback in improving AI tools, user experience, and addressing any limitations or challenges.
FAQs:
What is the Role of AI in Radiology?:
Explain the key role of AI in radiology, highlighting its ability to analyze medical images, detect anomalies, and provide diagnostic insights.
How Does AI Improve Diagnostic Accuracy?:
Discuss how AI’s pattern recognition and data analysis capabilities can enhance diagnostic accuracy by identifying subtle abnormalities that might be missed by human radiologists.
Will AI Replace Radiologists?:
Address the common misconception that AI will replace radiologists and emphasize the collaborative nature of AI integration, where radiologists and AI work together.
What Are Some Successful Applications of AI in Radiology?:
Provide examples of successful AI applications in radiology, such as early cancer detection, brain anomaly identification, and automated reporting.
How Does AI Benefit Patient Care?:
Explain how AI benefits patient care by enabling earlier and more accurate diagnoses, personalized treatment plans, and improved overall efficiency in radiology workflows.
What Challenges Does AI Adoption Present?:
Discuss challenges like data quality, ethical considerations, and resistance to change that come with AI adoption in radiology.
How Can Radiologists Learn to Use AI Tools?:
Offer insights into how radiologists can acquire the necessary skills through training programs, workshops, and continuous learning opportunities.
What About Patient Privacy and Data Security?:
Address concerns related to patient privacy and data security, emphasizing the importance of compliance with regulations and best practices.
Can AI Tools Be Trusted?:
Discuss how AI tools can be trusted through rigorous validation, transparent algorithms, and human oversight in the decision-making process.
What’s the Future of AI in Radiology?:
Provide insights into the evolving landscape of AI in radiology, including advancements in technology, expanded applications, and continued collaboration with radiologists.
Conclusion:
In conclusion, the integration of artificial intelligence (AI) into the realm of radiology marks a transformative era that holds immense promise for revolutionizing diagnostic capabilities. The synergy between human expertise and AI-driven insights presents opportunities for enhanced accuracy, efficiency, and personalized patient care. As AI-powered tools continue to evolve, radiologists are poised to benefit from streamlined workflows, improved anomaly detection, and the ability to provide more tailored treatment plans. However, as we embrace these advancements, it is crucial to address ethical considerations, ensure proper training, and maintain a collaborative approach, ultimately shaping a future where AI augments radiologists’ diagnostic prowess and contributes to better healthcare outcomes.