The field of pathology plays a pivotal role in healthcare by providing essential diagnostic insights through the examination of tissues and cells. With the advent of artificial intelligence (AI), a groundbreaking transformation is underway in pathology diagnostics. This exploration delves into how AI is revolutionizing traditional practices, offering the potential for faster, more accurate, and consistent diagnoses, ultimately enhancing patient care and redefining the landscape of medical decision-making.
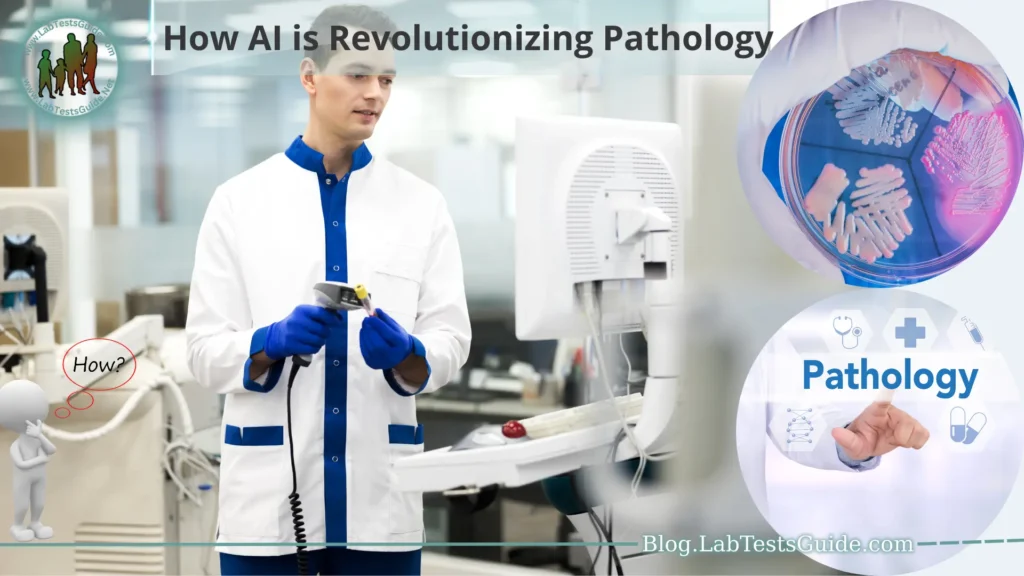
What Is Automated Diagnostics?
- Definition: Automated diagnostics refers to the utilization of artificial intelligence (AI) technologies to streamline and enhance the process of medical diagnosis. It involves the integration of AI algorithms and tools into the traditional diagnostic workflow.
- AI Applications: Automated diagnostics employ AI techniques, such as machine learning and deep learning, to analyze medical data, including images, scans, and test results. These AI systems can learn from large datasets to recognize patterns and anomalies that might not be readily apparent to human observers.
- Medical Imaging: One of the prominent applications of automated diagnostics is in medical imaging, where AI algorithms can analyze radiology images (X-rays, MRIs, CT scans) and histopathology slides with remarkable accuracy. This aids in the detection of abnormalities, tumors, and other conditions.
- Data Interpretation: AI-powered diagnostic systems can assist healthcare professionals in interpreting complex data by providing insights and suggestions. This can aid in identifying potential diagnoses and treatment options.
- Speed and Efficiency: By automating certain aspects of diagnosis, AI can significantly reduce the time required for analysis. This is particularly crucial in urgent cases where timely diagnosis can impact patient outcomes.
- Consistency and Standardization: Automated diagnostics help in maintaining consistent diagnostic criteria across different healthcare settings and even among different pathologists. This reduces variability in diagnoses and improves overall quality.
- Collaboration: AI systems in diagnostics act as tools to aid pathologists and healthcare providers, fostering collaboration between human experts and machine-driven insights. This collaborative approach can lead to more accurate and informed decisions.
- Learning and Improvement: AI algorithms can continuously learn and improve from new data, allowing them to adapt to emerging medical knowledge and improving diagnostic accuracy over time.
- Limitations and Human Oversight: While AI has shown remarkable potential, it’s important to note that these systems are not infallible. Human oversight remains critical to ensure that AI-generated suggestions align with clinical context and patient history.
- Ethical and Regulatory Considerations: The integration of AI in diagnostics raises ethical concerns about patient privacy, data security, and the potential for bias in algorithms. Regulatory bodies play a role in ensuring that AI systems meet certain standards before widespread clinical use.
- Future Directions: The field of automated diagnostics is evolving rapidly, and ongoing research aims to further enhance the capabilities of AI systems. This includes refining algorithms, addressing limitations, and expanding applications to various medical domains.
How to Implement AI-Powered Pathology Solutions:
- Assess Needs and Goals: Identify specific challenges and areas where AI could enhance pathology diagnostics, such as improving accuracy, speeding up analysis, or aiding in complex cases.
- Data Collection and Preparation: Gather a diverse and representative dataset of medical images, slides, and relevant patient data. Ensure data quality, consistency, and proper annotation for training AI algorithms.
- Choose AI Algorithms: Select suitable AI techniques based on the diagnostic task. For image analysis, convolutional neural networks (CNNs) are often used, while natural language processing (NLP) models can assist in text-based data.
- Collaboration with Pathologists: Involve pathologists and medical experts from the outset. Their insights are invaluable for refining AI algorithms, interpreting results, and ensuring clinical relevance.
- Development and Training: Develop or employ AI models using programming languages like Python and deep learning frameworks like TensorFlow or PyTorch. Train models using the prepared dataset, adjusting hyperparameters as needed.
- Validation and Testing: Validate the AI models using separate datasets to assess their accuracy, sensitivity, specificity, and other relevant metrics. Rigorous testing ensures reliable performance.
- Integration with Workflow: Develop a user-friendly interface that seamlessly integrates AI into the existing diagnostic workflow. This could be a standalone application or an enhancement to existing software.
- Interpretability and Explainability: Ensure that AI-generated results are interpretable by healthcare professionals. Techniques like attention maps for images or highlighting key factors for decision-making in reports can enhance trust.
- Regulatory Compliance: Depending on the jurisdiction, ensure that the AI-powered solution complies with medical device regulations and data privacy laws.
- Clinical Trials and Validation: Conduct clinical trials to validate the performance of the AI system in real-world scenarios. Compare AI-generated diagnoses with human experts’ assessments.
- Feedback and Iteration: Continuously gather feedback from pathologists and end-users. Use this feedback to refine and improve the AI algorithms, addressing any limitations or discrepancies.
Fundamentals of Pathology:
- Definition and Scope: Pathology is the study of the structural and functional changes caused by diseases in the body. It encompasses a wide range of disciplines, including anatomical pathology, clinical pathology, and molecular pathology.
- Disease Classification: Pathologists classify diseases based on their underlying causes, mechanisms, and characteristics. This classification aids in accurate diagnosis and treatment planning.
- Anatomical Pathology: This branch involves the examination of tissues and cells under a microscope to diagnose diseases. It includes surgical pathology (analysis of biopsies and surgical specimens) and cytopathology (study of individual cells).
- Clinical Pathology: Clinical pathologists analyze bodily fluids like blood, urine, and cerebrospinal fluid. They perform tests to diagnose conditions, monitor treatments, and assess overall health.
- Molecular Pathology: Molecular techniques are used to study the genetic and molecular changes associated with diseases. This includes identifying genetic mutations, gene expression patterns, and biomarkers.
- Diagnostic Techniques: Pathologists use a variety of techniques, including microscopy, immunohistochemistry, flow cytometry, and molecular assays, to detect and characterize diseases at the cellular and molecular levels.
- Diagnosis and Prognosis: Pathologists provide critical information for diagnosis, prognosis, and treatment decisions. Their insights help guide healthcare professionals in selecting appropriate therapies.
- Autopsy and Forensic Pathology: Pathologists conduct autopsies to determine the cause of death and contribute to forensic investigations. They analyze post-mortem tissue samples to understand the circumstances surrounding death.
- Research and Advancements: Pathology research contributes to a deeper understanding of disease mechanisms, leading to the development of new treatments, diagnostic tools, and therapies.
- Collaboration with Medical Teams: Pathologists work closely with other medical specialists, sharing their expertise to collectively diagnose and treat complex cases.
The Rise of AI in Healthcare:
- Introduction of AI: AI involves the development of computer systems that can perform tasks that typically require human intelligence, such as pattern recognition, decision-making, and learning from experience.
- Enhanced Diagnostics: AI algorithms excel in analyzing complex medical data, including images and scans, aiding in the early and accurate detection of diseases such as cancer, cardiovascular conditions, and neurological disorders.
- Medical Imaging Advancements: AI algorithms can process and interpret medical images (X-rays, MRIs, CT scans) with incredible accuracy, helping radiologists identify anomalies, tumors, and other subtle changes.
- Predictive Analytics: AI models can analyze patient data to predict disease outcomes, patient deterioration, and potential complications, enabling proactive interventions and personalized treatment plans.
- Drug Discovery and Development: AI accelerates drug discovery by analyzing vast datasets to identify potential drug candidates, predict their effectiveness, and optimize molecular structures.
- Personalized Medicine: AI analyzes genetic, molecular, and clinical data to tailor treatments based on individual patient profiles, optimizing effectiveness and minimizing adverse effects.
- Virtual Health Assistants: Chatbots and virtual assistants powered by AI provide patients with 24/7 access to medical information, symptom checking, appointment scheduling, and basic medical advice.
- Remote Monitoring and Telemedicine: AI-enabled devices monitor patients remotely, detecting deviations from normal health parameters and alerting healthcare providers for timely intervention.
- Administrative Efficiency: AI streamlines administrative tasks such as appointment scheduling, billing, and record-keeping, freeing up healthcare professionals to focus on patient care.
- Natural Language Processing (NLP): NLP enables AI systems to understand and generate human language, improving medical documentation, data extraction, and clinical notes.
- Ethical Considerations: The ethical use of AI in healthcare involves ensuring patient privacy, data security, transparency in algorithms, and addressing potential biases.
Advantages of AI in Pathology:
- Enhanced Accuracy: AI algorithms excel in pattern recognition, minimizing the risk of human error and enhancing diagnostic accuracy. They can identify subtle changes in images and slides that might be missed by human observers.
- Speed and Efficiency: AI-powered diagnostic tools can analyze large volumes of data in a fraction of the time it would take a human pathologist. This expedites diagnoses, especially in urgent cases.
- Consistency in Diagnoses: AI systems provide consistent results, reducing variability between different pathologists and ensuring standardized diagnostic criteria across various settings.
- Early Disease Detection: AI can identify early signs of diseases, allowing for timely intervention and treatment. This is particularly crucial for conditions with better prognosis when detected early.
- Handling Data Complexity: AI is capable of processing complex data, such as genomic sequences and molecular profiles, enabling a deeper understanding of diseases at the molecular level.
- Integrated Learning: AI algorithms continuously learn from new data, improving over time and adapting to emerging medical knowledge, leading to higher diagnostic accuracy.
- Support for Pathologists: AI doesn’t replace pathologists but acts as a valuable tool. It assists pathologists in making more informed decisions by highlighting relevant areas and potential diagnoses.
- Resource Allocation: By automating routine tasks like data analysis, AI frees up pathologists’ time to focus on more complex and critical cases, improving overall efficiency.
- Remote Consultations: AI-powered telepathology enables pathologists to collaborate and consult with experts worldwide, bridging geographical barriers and sharing expertise.
- Prediction and Prognosis: AI analyzes patient data to predict disease outcomes and assess the likelihood of treatment success, aiding in treatment planning and patient counseling.
AI Algorithms in Pathology:
- Enhanced Medical Imaging Analysis: AI algorithms excel in analyzing medical images, aiding in accurate disease diagnosis and identifying subtle anomalies that may be challenging for human pathologists.
- Diagnostic Assistance and Second Opinions: AI provides valuable diagnostic assistance by offering second opinions, suggesting potential diagnoses, and enhancing overall diagnostic accuracy.
- Tumor Detection and Early Cancer Diagnosis: AI algorithms accurately detect tumors in various medical images, contributing to early detection and diagnosis of cancerous growths.
- Predictive Disease Progression: By analyzing patient data, AI models predict disease progression, potential outcomes, and responses to different treatments, aiding in proactive patient management.
- Personalized Treatment Plans: AI identifies biomarkers linked to diseases, facilitating early detection and enabling tailored treatment plans based on individual patient profiles.
- Pattern Recognition for Complex Diseases: AI recognizes intricate patterns in data, assisting in the identification of diseases like Alzheimer’s, autoimmune disorders, and other complex conditions.
- Rapid Data Analysis and Reduced Waiting Times: AI’s rapid analysis of extensive datasets expedites the diagnostic process, minimizing patient waiting times and enhancing healthcare efficiency.
- Comprehensive Insights through Data Fusion: AI combines data from diverse sources, such as imaging and genomics, providing holistic insights for accurate diagnoses and treatment decisions.
- Region of Interest Segmentation: AI algorithms segment specific regions of interest in medical images, aiding pathologists in focusing on relevant areas during analysis.
- Digital Pathology Advancements: AI-enabled digitization of pathology slides streamlines analysis, storage, and sharing of digital repositories for improved collaboration and accessibility.
Integration of AI into Pathology Workflow:
- Assessment and Preparation: Identify areas where AI can assist in pathology. Gather diverse and relevant data for AI training.
- Algorithm Development: Select or develop appropriate AI algorithms. Train these algorithms using the collected data.
- Clinical Integration: Design user-friendly interfaces to present AI-generated insights to pathologists. Ensure AI results are explained in an understandable manner.
- Validation and Evaluation: Test the accuracy of AI using separate datasets. Evaluate the performance of AI with actual patient cases.
- Feedback Loop: Collect feedback from pathologists to improve and fine-tune AI algorithms.
- Ethical Considerations: Address concerns about patient data privacy and potential biases in AI algorithms.
- Regulatory Compliance: Ensure that AI aligns with medical regulations and standards.
- Scale and Deployment: Gradually introduce AI into the workflow, considering practicality and specific needs.
- Training and Support: Train pathologists to effectively interpret and utilize AI-generated insights.
- Monitoring and Continuous Improvement: Continuously monitor AI performance and update algorithms as required.
- Collaboration and Research: Collaborate with researchers to enhance the capabilities of AI in pathology.
- Benefits Communication: Highlight how AI contributes to improved patient outcomes and diagnostic accuracy.
- Scalability and Future Plans: Plan for future expansion of AI integration in pathology practice.
- Patient-Centric Approach: Emphasize the positive impact of AI on patient care and treatment.
- Continuous Learning: Stay informed about advancements in AI for ongoing improvement and innovation.
Future Prospects:
Healthcare and Medicine:
- Personalized Medicine: Tailoring treatments based on an individual’s genetic makeup and medical history.
- Genomic Medicine: Using genomic information to diagnose and treat diseases more precisely.
- Telemedicine Expansion: Continued growth of remote healthcare services for improved access and convenience.
- AI-Driven Drug Discovery: Accelerating the discovery of new drugs through AI-driven simulations and predictions.
- Medical Robotics: Integration of robots in surgeries and healthcare procedures for precision and minimally invasive procedures.
Artificial Intelligence:
- Explainable AI (XAI): Developing AI models that provide understandable explanations for their decisions.
- Ethical AI: Addressing bias, fairness, and transparency concerns in AI algorithms.
- AI in Creativity: Advancements in AI-generated art, music, and content creation.
- Quantum Computing: Harnessing the power of quantum computers for solving complex problems and accelerating AI research.
Space Exploration:
- Moon and Mars Missions: Continued missions to explore and establish a presence on the Moon and Mars.
- Private Space Travel: Increasing accessibility to space with private companies offering commercial spaceflights.
- Astrobiology: Searching for signs of life on other planets and moons within our solar system.
Renewable Energy and Environment:
- Solar Energy Advancements: Improved solar panels, storage solutions, and efficiency.
- Battery Technology: Developing better and more sustainable energy storage options.
- Carbon Capture and Climate Solutions: Innovations to combat climate change and reduce carbon emissions.
Automation and Robotics:
- Autonomous Vehicles: Progress in self-driving cars and drones for safer and more efficient transportation.
- Industry 4.0: Further integration of automation and IoT in manufacturing and supply chains.
- Healthcare Robotics: Growing role of robots in assisting surgeries, rehabilitation, and patient care.
Biotechnology:
- CRISPR Technology: Advancing gene-editing techniques for potential treatment of genetic diseases.
- Synthetic Biology: Engineering organisms for various applications, from medicine to biofuels.
FAQs:
What is AI-powered pathology?
AI-powered pathology involves the integration of artificial intelligence algorithms and technologies into the field of pathology. It aims to enhance diagnostic accuracy, speed up analysis, and improve patient outcomes.
How does AI assist pathologists in diagnosis?
AI assists pathologists by analyzing medical images and data to identify patterns, anomalies, and potential disease markers. It provides second opinions, aids in tumor detection, and offers predictive insights for disease progression.
Is AI replacing pathologists?
No, AI is not replacing pathologists. It acts as a supportive tool, helping pathologists make more accurate and informed decisions. Pathologists retain their expertise in interpreting complex medical information.
What are the benefits of AI in pathology?
AI enhances diagnostic accuracy, speeds up analysis, supports early disease detection, assists in personalized treatment planning, and contributes to more efficient healthcare processes.
How is patient data privacy ensured when using AI in pathology?
AI solutions must adhere to strict data privacy regulations and security measures. Patient data is anonymized, encrypted, and only accessible to authorized personnel.
Can AI algorithms be biased in pathology diagnosis?
Yes, AI algorithms can inherit biases from the data they are trained on. Efforts are made to mitigate bias by using diverse and representative datasets and applying fairness-aware algorithms.
What role does AI play in medical imaging analysis?
AI algorithms, particularly convolutional neural networks (CNNs), excel in analyzing medical images like X-rays, MRIs, and histopathology slides. They assist in identifying anomalies and patterns associated with diseases.
How is AI integrated into the pathology workflow?
AI integration involves identifying needs, selecting or developing algorithms, validating their accuracy, creating user-friendly interfaces, and continuously refining the algorithms based on pathologist feedback.
Are there any ethical concerns with AI in pathology?
Ethical considerations include patient data privacy, algorithm transparency, fairness, and accountability. Efforts are made to ensure AI benefits outweigh risks and that patient care remains the priority.
What does the future hold for AI in pathology?
The future of AI in pathology includes enhanced diagnostic accuracy, improved patient care, expanded use of AI-powered tools, and continued collaborations between AI and human experts.
Conclusion:
In conclusion, the integration of artificial intelligence (AI) into pathology marks a transformative advancement in healthcare diagnostics. By enhancing the capabilities of pathologists through accurate image analysis, predictive insights, and personalized treatment recommendations, AI is poised to revolutionize disease detection and patient care. While AI complements the expertise of pathologists, it does not replace them; rather, it empowers them with efficient tools for more precise diagnoses. Ethical considerations, ongoing collaboration, and the responsible implementation of AI ensure that the future of pathology remains patient-centered, innovative, and built upon the synergy between human expertise and technological innovation.