Explore the transformative synergy of AI and mental health, uncovering innovative tools for early detection, intervention, and support. Discover how artificial intelligence is revolutionizing the landscape of mental wellness, offering proactive solutions to identify and address challenges for improved emotional wellbeing.
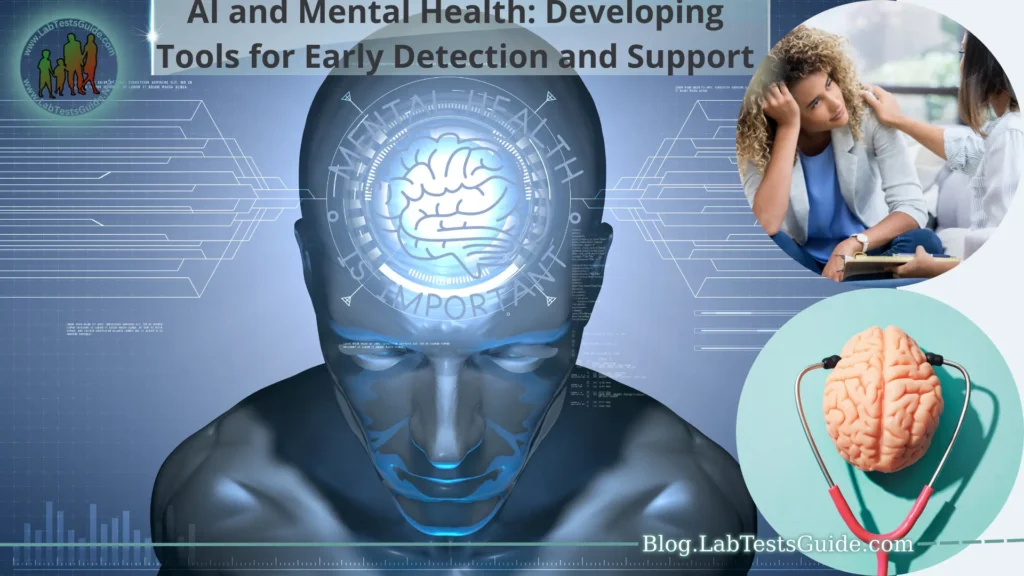
What Is the Role of AI in Enhancing Early Detection and Support for Mental Health?
Data Analysis and Pattern Recognition:
- AI analyzes vast datasets to identify subtle patterns in language, behavior, and emotions.
- Detection of deviations from baseline behavior aids in recognizing potential mental health issues.
Predictive Analytics:
- AI algorithms process diverse data sources to predict the onset of mental health conditions.
- Early identification enables timely interventions and personalized treatment strategies.
Chatbots and Virtual Assistants:
- AI-powered chatbots provide immediate, accessible support to individuals in distress.
- Text or voice interactions offer coping strategies, resources, and a nonjudgmental presence.
Personalized Treatment Plans:
- AI assists mental health professionals in tailoring treatment plans to individual needs.
- Analysis of responses to interventions helps refine strategies for optimal outcomes.
Neuroimaging and Biomarker Analysis:
- AI processes neuroimaging data to identify brain activity patterns linked to mental health conditions.
- Analysis of biological markers aids in understanding susceptibility and potential diagnoses.
Emotion Recognition and Physiological Signals:
- AI interprets facial expressions, vocal tones, and physiological cues to infer emotional states.
- Remote monitoring detects mood shifts and prompts timely interventions.
Therapist Support Tools:
- AI provides insights during therapy sessions by analyzing conversation dynamics.
- Feedback helps therapists refine their approaches and enhance the therapeutic process.
Digital Therapeutics and Self-Help Apps:
- AI-driven apps deliver evidence-based therapeutic exercises and techniques.
- Increases accessibility to mental health support and encourages proactive self-care.
Ethical Considerations:
- Ensuring data privacy and adhering to ethical guidelines in collecting and using sensitive data.
- Striking a balance between technology-driven insights and individual rights.
Collaboration for Holistic Care:
- AI complements, rather than replaces, human care and expertise.
- Collaboration among mental health professionals, AI developers, and policymakers is crucial.
How to Implement AI Tools for Early Detection and Support in Mental Health:
Needs Assessment:
- Identify the specific mental health conditions you aim to address and the target population.
- Understand the existing challenges in early detection and support within the context.
Data Collection and Preparation:
- Gather diverse datasets, including text, audio, and physiological data, with appropriate consent.
- Ensure data privacy and comply with ethical guidelines during data collection and storage.
Algorithm Development:
- Collaborate with data scientists and AI experts to develop algorithms for pattern recognition and predictive analytics.
- Train models using labeled data and consider using pre-trained models if applicable.
Integration with Existing Systems:
- Integrate AI tools with existing mental health platforms, electronic health records, or therapy systems.
- Ensure seamless user experience and data flow between AI tools and other resources.
Chatbot or Virtual Assistant Creation:
- Develop a user-friendly chatbot or virtual assistant capable of offering immediate support and resources.
- Implement natural language processing (NLP) to enable effective communication.
Personalization and User Profiling:
- Design AI systems that adapt to individual user needs and preferences over time.
- Use user feedback and interaction history to tailor support and interventions.
Therapist Collaboration:
- Collaborate with mental health professionals to validate the effectiveness of AI tools.
- Gather insights from therapists’ experiences to refine and improve the tools.
Ethical Guidelines and Privacy Protection:
- Develop strict ethical guidelines for data usage and sharing.
- Ensure users’ privacy and informed consent are maintained throughout the process.
Testing and Validation:
- Conduct thorough testing and validation of AI tools with diverse user groups.
- Gather feedback to identify any technical or usability issues.
Continuous Improvement:
- Regularly update and fine-tune AI models to improve accuracy and relevance.
- Stay updated on the latest advancements in AI and mental health research.
AI-Powered Support Tools:
Chatbots and Virtual Assistants:
- AI chatbots engage in text or voice-based conversations, offering immediate support and resources.
- They can provide coping strategies, relaxation techniques, and information about mental health conditions.
- Conversations can be tailored based on user inputs and language patterns.
Emotion Tracking Apps:
- AI-powered apps track users’ emotions through self-reported data, facial expression analysis, or physiological signals.
- Users receive insights into their emotional well-being and recommendations for managing stress and mood.
Crisis Hotline Chatbots:
- AI chatbots offer real-time assistance to individuals in crisis, providing empathetic responses and directing users to appropriate resources.
- They can help de-escalate distressing situations and encourage users to seek professional help.
Mood and Journaling Apps:
- AI tools analyze users’ journal entries and mood logs to identify patterns and triggers.
- Insights can help users gain self-awareness and work on strategies for emotional regulation.
Digital Therapeutics Platforms:
- AI-driven platforms deliver evidence-based therapeutic interventions, such as cognitive-behavioral therapy (CBT) exercises.
- They provide users with structured modules to manage symptoms and improve mental well-being.
Virtual Reality (VR) Therapy:
- AI-powered VR experiences simulate therapeutic environments, helping users confront and manage anxiety or phobias.
- The AI tailors the experience based on user progress and reactions.
Voice Analysis Tools:
- AI analyzes vocal patterns to detect emotional states, stress levels, and changes in mood.
- This technology can be used for real-time emotional monitoring and interventions.
Medication Adherence Apps:
- AI-powered apps remind users to take medications, track adherence, and provide education about prescribed treatments.
- They can send notifications and offer support to promote medication compliance.
Self-Help Resource Recommenders:
- AI algorithms recommend self-help articles, videos, podcasts, and other resources based on users’ needs and preferences.
- These tools empower users with educational materials for self-improvement.
User Engagement and Feedback Analysis:
- AI tools analyze user interactions with mental health apps to understand engagement levels and identify areas for improvement.
- Feedback loops help developers refine the tools and enhance their effectiveness.
Technological Applications:
Neuroimaging and Brain Activity Analysis:
- Functional Magnetic Resonance Imaging (fMRI) and Electroencephalography (EEG) data are processed using AI algorithms to identify patterns associated with mental health disorders.
- Brain activity analysis helps in understanding the neural basis of conditions like depression, schizophrenia, and anxiety.
Predictive Analytics and Machine Learning:
- Machine learning models analyze large datasets to predict the likelihood of developing mental health conditions.
- Early identification of risk factors helps in implementing preventive interventions and support.
Natural Language Processing (NLP) for Text Analysis:
- NLP algorithms analyze text data from various sources, such as social media posts and clinical notes, to identify linguistic markers of mental health issues.
- Text analysis assists in early detection and monitoring of mood changes and emotional well-being.
Emotion Recognition and Analysis:
- AI systems analyze facial expressions, vocal intonations, and physiological signals to infer emotional states.
- Emotion recognition technology aids in understanding user emotions and providing appropriate support.
Mobile Apps and Wearables:
- Mobile apps and wearable devices track users’ activity, sleep patterns, and physiological responses.
- Data from these devices offer insights into daily routines and help monitor fluctuations in mental health.
Virtual Reality (VR) and Augmented Reality (AR):
- VR and AR technologies create immersive environments for exposure therapy and relaxation techniques.
- They offer controlled settings to address anxiety, phobias, and stress.
Teletherapy and Remote Monitoring:
- Video conferencing and teletherapy platforms enable remote counseling sessions between therapists and clients.
- Remote monitoring of users’ progress and emotional well-being facilitates ongoing support.
Cognitive Training and Brain-Computer Interfaces (BCIs):
- BCIs allow users to control devices using their brain activity, aiding individuals with conditions like ADHD or neurodegenerative disorders.
- Cognitive training exercises enhance cognitive functions and support mental health.
Genetic and Biomarker Analysis:
- AI analyzes genetic data and biomarkers to identify genetic predispositions to mental health conditions.
- Biomarker analysis assists in personalized treatment planning and early intervention.
Personalized Treatment Recommendations:
- AI algorithms process individual health records, treatment history, and response data to recommend personalized treatment plans.
- Personalization optimizes treatment outcomes by tailoring interventions to each individual.
Ethical and Privacy Considerations:
Informed Consent:
- Obtain clear and informed consent from users before collecting their data or involving them in AI-driven interventions.
- Clearly explain the purpose, risks, and benefits of using AI tools in mental health support.
Data Privacy and Security:
- Implement robust security measures to protect user data from unauthorized access, breaches, and cyber threats.
- Utilize encryption and other safeguards to ensure data integrity and confidentiality.
Anonymization and De-Identification:
- Anonymize or de-identify user data to prevent the identification of individuals when conducting data analysis or sharing findings.
User Control and Transparency:
- Provide users with control over their data, including the ability to review, modify, or delete their information.
- Offer transparent explanations of how AI tools work, what data is collected, and how it will be used.
Bias and Fairness:
- Address biases in AI algorithms to ensure that predictions and recommendations are not unfairly influenced by factors such as race, gender, or socioeconomic status.
- Regularly audit and assess algorithms for potential bias and discrimination.
Professional Oversight:
- Collaborate with mental health professionals to ensure that AI tools enhance, rather than replace, human care.
- Maintain a clear distinction between AI-driven support and the expertise provided by trained therapists.
User Empowerment:
- Empower users by clearly communicating the limitations of AI tools and encouraging them to seek professional help when necessary.
- Offer guidance on interpreting AI-generated insights and suggestions.
Sensitive Data Handling:
- Handle sensitive information related to mental health diagnoses, treatment, and experiences with the utmost care and discretion.
- Comply with legal and ethical guidelines for handling health-related data.
Long-Term Effects and Accountability:
- Consider the potential long-term effects of AI interventions on users’ mental health and well-being.
- Establish mechanisms for accountability and address any unintended consequences that may arise.
Collaboration and Future Directions:
Interdisciplinary Partnerships:
- Foster collaboration between AI experts, data scientists, psychologists, psychiatrists, therapists, and social workers.
- Combine technical expertise with clinical insights to develop AI tools that are clinically effective and ethically sound.
User-Centered Design:
- Involve individuals with lived experiences of mental health challenges in the design and development process.
- Gather user feedback to ensure AI tools are user-friendly, culturally sensitive, and aligned with real-world needs.
Clinical Validation and Integration:
- Collaborate with mental health professionals to validate the efficacy of AI tools through rigorous clinical trials and studies.
- Integrate AI tools seamlessly into existing mental health care systems to enhance patient outcomes.
Ethical Review and Oversight:
- Establish multidisciplinary ethical review boards to evaluate the deployment of AI in mental health and ensure compliance with ethical guidelines.
- Involve ethicists, privacy experts, and legal professionals to provide guidance on responsible implementation.
Data Sharing and Research Collaboration:
- Facilitate data sharing across institutions and jurisdictions to improve AI model training and validation.
- Collaborate on research initiatives to advance the understanding of AI’s impact on mental health outcomes.
Policy and Regulation:
- Collaborate with policymakers to develop regulations and guidelines that promote the ethical and responsible use of AI in mental health.
- Advocate for policies that ensure data privacy, transparency, and accountability.
Longitudinal Studies and Outcome Tracking:
- Collaboratively design long-term studies to assess the impact of AI tools on mental health outcomes over time.
- Track user experiences and evaluate the effectiveness of interventions.
Global Reach and Accessibility:
- Collaborate with international organizations to address cultural and linguistic diversity in AI-powered mental health support.
- Work towards making AI tools accessible and relevant to diverse populations globally.
Addressing Disparities:
- Collaborate on initiatives to address disparities in access to AI-powered mental health support, considering socioeconomic, geographic, and demographic factors.
Continual Learning and Adaptation:
- Foster a culture of continuous learning and adaptation through knowledge-sharing, conferences, workshops, and interdisciplinary forums.
- Stay informed about emerging AI advancements and adapt AI tools accordingly.
Case Studies and Success Stories:
Woebot:
- Woebot is an AI-powered chatbot designed to provide cognitive-behavioral therapy (CBT) interventions.
- It engages users in conversations, offers coping strategies, and guides them through structured exercises.
- Research has shown that Woebot can significantly reduce symptoms of depression and improve mood.
Affectiva’s Emotion Recognition:
- Affectiva’s AI technology analyzes facial expressions and vocal tones to infer users’ emotional states.
- It has been used to detect signs of stress, anxiety, and depression in real-time.
- Applications range from monitoring mental health during therapy sessions to assessing emotional responses to marketing content.
Mindstrong Health:
- Mindstrong Health’s platform uses smartphone data to passively monitor users’ digital behavior, including typing patterns and screen interactions.
- The AI analyzes these patterns to detect early signs of mood changes and mental health fluctuations.
- This approach shows promise in providing objective and continuous mental health tracking.
Talkspace and AI-Assisted Therapy:
- Talkspace integrates AI-assisted therapy with human therapists to offer personalized support.
- AI algorithms analyze user messages and therapist responses to offer suggestions for therapist interventions.
- This hybrid model enhances therapeutic outcomes and extends the reach of mental health care.
VR Therapy for PTSD:
- Virtual reality (VR) exposure therapy has been used to treat post-traumatic stress disorder (PTSD).
- VR environments recreate traumatic situations in a controlled setting, allowing individuals to confront and process their emotions.
- Studies have shown promising results in reducing PTSD symptoms and improving emotional well-being.
Mindstrong and Smartphone Data:
- Mindstrong uses smartphone data, such as typing speed, social interactions, and sleep patterns, to monitor mental health.
- AI analyzes this data to detect changes indicative of mood disorders.
- The approach offers a non-intrusive and continuous way to track mental well-being.
SPARX Video Game for Depression:
- SPARX is an interactive video game designed to deliver CBT to adolescents with depression.
- Players engage with virtual characters and complete challenges that teach coping skills.
- Clinical trials have shown SPARX to be as effective as traditional therapy in reducing symptoms of depression.
Ginger’s AI-Powered Coaching:
- Ginger offers an AI-powered mental health coaching app that provides on-demand support and interventions.
- Users can have text-based conversations with AI coaches, addressing stress, anxiety, and other concerns.
- The app complements traditional therapy and promotes mental well-being.
Challenges and Limitations:
Data Privacy and Security Concerns:
- Collecting and analyzing sensitive mental health data raises concerns about user privacy and data security.
- Unauthorized access, breaches, and misuse of data could have severe consequences for individuals’ well-being and trust in AI technologies.
Ethical Considerations and Bias:
- AI algorithms can inherit biases present in training data, leading to unfair or inaccurate predictions.
- Ensuring fairness, transparency, and ethical use of AI in mental health is essential to avoid reinforcing existing inequalities.
Human-AI Interaction:
- Striking the right balance between AI-driven support and human interaction is crucial.
- Overreliance on AI tools could potentially lead to dehumanization of mental health care and hinder the therapeutic relationship.
Lack of Personalization and Context:
- AI tools may struggle to understand the nuances of an individual’s mental health journey and personal circumstances.
- The one-size-fits-all approach might not address unique needs and cultural sensitivities.
- Regulatory and Legal Challenges: The rapidly evolving nature of AI technology can outpace regulatory frameworks, leading to uncertainty about data usage, liability, and accountability.
User Acceptance and Engagement:
- Some individuals might be hesitant to engage with AI tools for mental health support due to concerns about privacy, trust, or unfamiliarity with technology.
- Complexity of Mental Health Conditions: Many mental health conditions are multifaceted and complex, making it challenging for AI to provide accurate diagnoses or treatment recommendations.
Human Oversight and Misinterpretation:
- Misinterpretation of user inputs or AI-generated insights could lead to inappropriate interventions or advice.
- Human oversight is crucial to ensure AI recommendations align with users’ needs.
Limited Access and Digital Divide:
- Not all individuals have access to the necessary technology or internet connectivity required for AI-powered mental health support.
- This can exacerbate existing disparities in mental health care access.
- Unintended Consequences: Relying solely on AI tools could potentially depersonalize mental health care and discourage individuals from seeking face-to-face professional help.
FAQs:
What is the role of AI in mental health?
AI plays a significant role in mental health by analyzing data, identifying patterns, and providing personalized interventions to enhance early detection, treatment, and support for individuals with mental health conditions.
How does AI contribute to early detection of mental health issues?
AI analyzes language, behavior, and physiological data to identify subtle changes that may indicate the onset of mental health issues, enabling timely intervention and support.
What are AI-powered support tools in mental health?
AI-powered support tools include chatbots, virtual assistants, emotion recognition, therapy apps, and virtual reality experiences designed to offer immediate assistance, coping strategies, and interventions to individuals in need.
Are AI-powered mental health tools effective?
Research suggests that AI-powered tools can be effective in enhancing mental health support. They can provide valuable insights, increase accessibility, and offer personalized interventions.
How do AI chatbots provide mental health support?
AI chatbots engage in text or voice conversations, offering users a nonjudgmental space to express their emotions, providing coping techniques, and suggesting resources for mental health well-being.
What ethical considerations should be taken into account when using AI in mental health?
Ethical considerations include data privacy, bias mitigation, user consent, transparency, fair algorithms, accountability, and ensuring AI tools complement human care rather than replace it.
Can AI tools replace traditional mental health professionals?
AI tools are designed to complement, not replace, traditional mental health professionals. They provide support and resources but lack the human understanding and empathy essential for therapeutic relationships.
How do AI tools handle user privacy and data security?
Responsible AI tools prioritize user privacy through secure data storage, anonymization, encryption, and adherence to data protection regulations to prevent unauthorized access or breaches.
What challenges does AI face in mental health applications?
Challenges include data privacy concerns, ethical considerations, biases in algorithms, human-AI interaction, lack of personalization, regulatory issues, and ensuring user engagement.
How can collaboration between AI developers and mental health professionals benefit users?
Collaboration ensures that AI tools are clinically validated, ethically developed, and meet the real-world needs of individuals with mental health challenges.
Conclusion:
In conclusion, the integration of AI tools for early detection and support in mental health represents a promising advancement in the field, offering new avenues to improve the well-being of individuals facing mental health challenges. The convergence of technological innovation, data analysis, and clinical expertise has the potential to transform how mental health care is delivered, making it more accessible, personalized, and effective.