The concept of AI-powered drug discovery as a game-changer in pharmaceutical research and development, promising to significantly expedite the process of identifying and developing new therapeutic solutions.
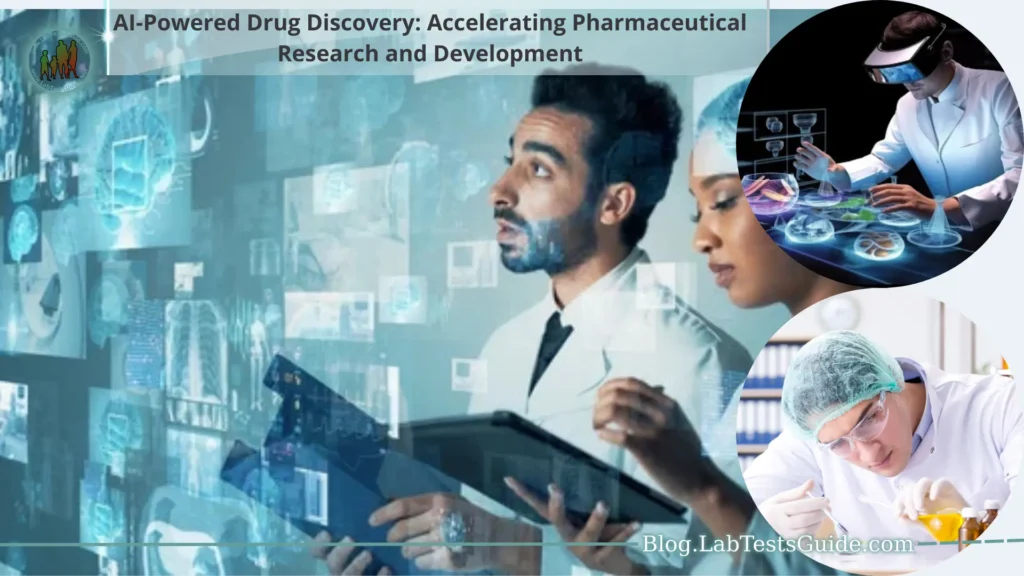
What is AI-Powered Drug Discovery?
- Definition: AI-Powered Drug Discovery refers to the integration of artificial intelligence (AI) technologies into the process of identifying, designing, and developing new pharmaceutical compounds and therapies.
- Role of AI: It involves the utilization of advanced machine learning, data analysis, and predictive modeling techniques to enhance various stages of drug discovery, from target identification to clinical trial optimization.
- Data Analysis: AI extracts valuable insights from massive datasets, including genomics, proteomics, chemical libraries, and clinical data, enabling researchers to make more informed decisions.
- Predictive Modeling: AI algorithms predict drug-target interactions, analyze compound properties, and identify potential leads, significantly expediting the search for promising candidates.
- Virtual Screening: AI-driven virtual screening involves sifting through vast compound databases to identify molecules with potential therapeutic properties, saving time and resources compared to traditional methods.
- Target Identification: AI aids in identifying disease-related molecular targets by analyzing biological data, thus improving the accuracy of target selection for drug development.
- Clinical Trial Optimization: AI optimizes clinical trial design by analyzing patient data to identify suitable participants, enhancing trial efficiency and reducing costs.
- Drug Repurposing: AI identifies existing drugs that could be repurposed for new therapeutic uses, providing faster paths to treatment options.
- Safety Prediction: AI models predict potential drug toxicities and adverse effects, allowing researchers to mitigate safety risks early in the development process.
- Regulatory Approval: AI-generated insights contribute to regulatory submissions, enhancing the credibility and efficiency of the drug development process.
- Ethical Considerations: As AI influences decision-making, addressing ethical concerns related to data privacy, bias, and transparency becomes crucial.
- Innovation Catalyst: AI-Powered Drug Discovery has the potential to revolutionize the pharmaceutical industry, accelerating the development of life-saving treatments and improving patient outcomes.
How to Leverage AI for Drug Discovery:
- Data Collection and Integration: Gather diverse data sources and integrate them for analysis.
- Machine Learning Algorithms: Select appropriate machine learning models for drug-target interactions and compound properties.
- Virtual Screening and Compound Design: Use AI-driven virtual screening to identify potential drug candidates.
- Target Identification and Validation: Apply AI to analyze biological data and validate disease-related molecular targets.
- Clinical Trial Optimization: Optimize clinical trial design and participant selection using AI.
- Drug Repurposing and Combination Therapy: Identify new therapeutic uses for existing drugs and design combination therapies.
- Safety Assessment: Develop AI models to predict drug-induced toxicities.
- Regulatory Compliance: Ensure AI results meet regulatory standards.
- Ethical Considerations: Address ethical challenges in AI-powered drug discovery.
- Collaboration and Expertise: Foster interdisciplinary collaboration between AI and domain experts.
- Continuous Learning: Stay updated with advancements in AI and drug discovery.
- Validation and Testing: Validate AI predictions through experiments and testing.
Data Collection and Preprocessing:
- Comprehensive Data Gathering: Collect a wide range of data sources, including genomics, proteomics, chemical information, and clinical records.
- Data Quality Enhancement: Apply data preprocessing techniques to clean and normalize data, addressing inconsistencies and errors.
- Integration and Standardization: Integrate different data types and formats to create a unified dataset for analysis.
- Feature Engineering: Extract relevant features from raw data to enhance the effectiveness of AI models.
- Handling Missing Data: Develop strategies to deal with missing data points, ensuring robustness of AI algorithms.
- Data Augmentation: Enhance dataset size through techniques like duplication, interpolation, and generation.
- Normalization and Scaling: Normalize data distributions and scale features to ensure fair comparisons and model stability.
- Dimensionality Reduction: Employ techniques like principal component analysis (PCA) to reduce data dimensionality while retaining crucial information.
- Quality Control: Implement validation checks to ensure data accuracy, consistency, and adherence to research goals.
- Data Privacy and Security: Adhere to ethical guidelines and data protection regulations while handling sensitive patient information.
Machine Learning and Predictive Modeling:
- Algorithm Selection: Choose appropriate machine learning algorithms based on the nature of the problem, such as classification, regression, or clustering.
- Training Data Preparation: Split the dataset into training, validation, and testing sets for model training and evaluation.
- Feature Selection and Engineering: Identify relevant features that contribute to accurate predictions and engineer new features when necessary.
- Hyperparameter Tuning: Optimize algorithm parameters to achieve the best model performance through techniques like grid search or random search.
- Model Training: Train the selected machine learning models on the training dataset to learn patterns and relationships.
- Model Evaluation: Assess model performance using appropriate metrics such as accuracy, precision, recall, F1-score, or area under the curve (AUC).
- Overfitting and Regularization: Address overfitting by applying regularization techniques like L1, L2 regularization or dropout.
- Ensemble Learning: Combine multiple models to improve overall prediction accuracy and generalization.
- Interpretable Models: Choose interpretable models when transparency and explainability are crucial for decision-making.
- Model Validation: Validate model results using cross-validation and holdout datasets to ensure robustness and generalization.
- Model Deployment: Deploy trained models to real-world scenarios, considering factors like scalability, efficiency, and integration.
Target Identification and Validation:
- Biological Data Analysis: Utilize AI to analyze genomics, proteomics, and other biological data to identify potential disease-related molecular targets.
- Network Analysis: Employ network-based approaches to uncover interactions and relationships within biological systems.
- Functional Annotation: Assign functions to genes and proteins to understand their roles in disease pathways.
- Pathway Analysis: Explore signaling pathways and biological processes to identify key nodes and targets.
- Drug Target Prioritization: Rank and prioritize potential targets based on their relevance to the disease and druggability.
- Validation Strategies: Employ experimental methods to validate predicted targets, including knockdown studies, functional assays, and expression analyses.
- Omics Data Integration: Combine multiple omics datasets to enhance target validation accuracy and reliability.
- Biomarker Identification: Identify biomarkers associated with disease progression and treatment response to guide target validation.
- Clinical Data Correlation: Correlate target expression and activity with clinical outcomes to validate their therapeutic relevance.
- Feedback Loop: Iteratively refine target selection and validation based on new insights and data.
Clinical Trial Optimization:
- Patient Recruitment Enhancement: Utilize AI to identify potential trial participants from diverse sources, including electronic health records and patient databases.
- Predictive Analytics: Develop models that predict patient enrollment rates and trial completion times, aiding in resource allocation.
- Trial Site Selection: Analyze geographical and demographic data to identify optimal locations for trial sites.
- Protocol Design Optimization: Use AI insights to refine trial protocols, enhancing participant experience and data collection.
- Adaptive Trials: Implement adaptive trial designs that can be modified in response to incoming data, maximizing efficiency.
- Real-world Evidence Integration: Incorporate real-world evidence data to supplement clinical trial results and enhance the understanding of treatment effects.
- Patient Stratification: Apply AI to stratify patient populations, identifying subgroups that might respond differently to treatments.
- Outcome Prediction: Predict trial outcomes based on patient characteristics, treatment protocols, and historical data.
- Safety Monitoring: Develop AI algorithms to monitor patient safety data in real-time, ensuring rapid detection of adverse events.
- Data Quality Enhancement: Employ AI to ensure data accuracy, consistency, and compliance throughout the trial.
Drug Repurposing and Combination Therapy:
- Existing Drug Exploration: Employ AI to analyze drug databases and identify potential new uses for existing approved drugs.
- Pharmacological Similarity Analysis: Use AI to assess the similarity between compounds to predict repurposing opportunities.
- Biological Network Analysis: Analyze biological networks to uncover connections between drugs and disease pathways.
- Clinical Data Integration: Combine clinical data to identify patterns suggesting alternative therapeutic indications.
- Side Effect Prediction: Predict potential side effects of repurposed drugs using AI models.
- Combination Therapy Simulation: Use AI to simulate interactions between multiple drugs and predict synergistic effects.
- Optimal Dose Identification: Employ AI to identify appropriate dosages for combination therapies to maximize efficacy.
- Patient Stratification: Stratify patients based on molecular profiles to personalize combination therapies.
- Predicting Drug Interactions: Analyze potential interactions between drugs to avoid adverse effects in combination.
- Preclinical Validation: Validate repurposed drugs and combination therapies using preclinical models.
Regulatory Considerations and Ethical Challenges:
- Regulatory Acceptance: Address regulatory guidelines for incorporating AI-generated insights into drug discovery and development.
- Validation and Transparency: Develop methods to validate AI models and ensure transparency in their decision-making processes.
- Data Privacy and Security: Implement measures to protect patient data and ensure compliance with data privacy regulations.
- Bias and Fairness: Mitigate bias in AI models to avoid perpetuating disparities in drug development and healthcare.
- Interpretability and Explainability: Create interpretable AI models to explain how decisions are made, improving regulatory scrutiny.
- Human Oversight: Maintain human oversight in critical decision points, ensuring AI-generated results align with human expertise.
- Data Source Reliability: Validate the reliability and credibility of data sources used to train AI models.
- Ethical AI Use: Adhere to ethical guidelines in AI research, addressing issues of bias, accountability, and social impact.
- Translational Challenges: Bridge the gap between AI-driven discovery and traditional drug development processes, meeting regulatory standards.
- Collaboration with Regulators: Foster collaboration between AI researchers, pharmaceutical companies, and regulatory agencies to establish best practices.
FAQs:
What is AI-powered drug discovery?
AI-powered drug discovery involves utilizing artificial intelligence techniques to expedite and enhance various stages of pharmaceutical research and development.
How does AI contribute to drug discovery?
AI aids in data analysis, predictive modeling, target identification, safety prediction, and clinical trial optimization, leading to faster and more accurate insights.
What data sources are used in AI-driven drug discovery?
Genomics, proteomics, chemical databases, clinical records, and real-world evidence are some of the diverse data sources utilized.
Can AI predict drug-target interactions?
Yes, AI models can predict potential interactions between drugs and molecular targets, aiding in lead identification.
What is virtual screening, and how does AI help?
Virtual screening uses computational methods to identify potential drug candidates. AI accelerates this process by efficiently analyzing vast compound libraries.
How does AI assist in clinical trial optimization?
AI predicts patient recruitment rates, optimizes trial site selection, and refines trial protocols, leading to efficient and successful trials.
What is drug repurposing? How does AI play a role?
Drug repurposing involves finding new uses for existing drugs. AI analyzes data to identify drugs that could be effective for different indications.
What ethical challenges does AI pose in drug discovery?
Ethical concerns include data privacy, bias in AI models, transparency, and the potential impact on patient care.
Can AI models be trusted for regulatory submissions?
AI-generated insights need to be rigorously validated and aligned with regulatory standards to gain acceptance.
How can AI and human expertise coexist in drug discovery?
Human oversight and collaboration with AI are essential to ensure that AI-generated results align with domain knowledge and ethical considerations.
Conclusion:
In conclusion, the integration of artificial intelligence into drug discovery stands poised to redefine the landscape of pharmaceutical research and development. AI-powered methodologies, from data analysis to predictive modeling, offer unprecedented insights, enabling the rapid identification of promising drug candidates, optimization of clinical trials, and innovative approaches to repurposing and combination therapies. While AI’s transformative potential is evident, it also brings forth regulatory and ethical considerations that necessitate careful navigation. The collaborative synergy between AI-driven innovation and human expertise promises to usher in a new era of accelerated drug discovery, ultimately translating into more effective treatments and improved patient outcomes.